For one of our customers, the number one intermediary for financial products servicing cars and utility vehicles as well as a member of one of Poland’s largest media groups, the cost of posting was relatively high. Our customer used to post several thousand offers on the top ecommerce car sale platform which required a lot of manual preparation, leading to high costs. As the number of offers being posted grew, monitoring and maintaining their effectiveness became a serious operational challenge.
Optimising eCommerce CPC
Challenge
Solution
We recommended that the data gathered in the customer’s data lake be used to train machine learning models that would predict the performance of each offer posted.
Our team was already familiar with the data and our customer’s environment as we had previously cooperated to help our customer to become a more data-oriented company (you can read about it here). As a result, the most time-consuming part of our work, i.e. data understanding and analysis, could be greatly reduced and in some areas skipped over, which resulted in a much quicker delivery.
Our data scientists used several million data points from our customer’s historical offers posted to develop a model that predicts the number of clicks on a particular offering after 3 days as well as 7 days. Input data used to make predictions consisted of characterising the subject of the post, such as automaker brand and model, as well as more general environmental characterizations, such as day of the week and time of posting. The project was implemented using BRAINHINT’s AI Farm software installed on Azure Cloud.
The number of potential combinations of input parameters was limited to tens of thousands, thus predictions were made for every combination of the parameters after each training session of the model. Results were saved into a database and presented in the form of an interactive report in Microsoft’s PowerBI.
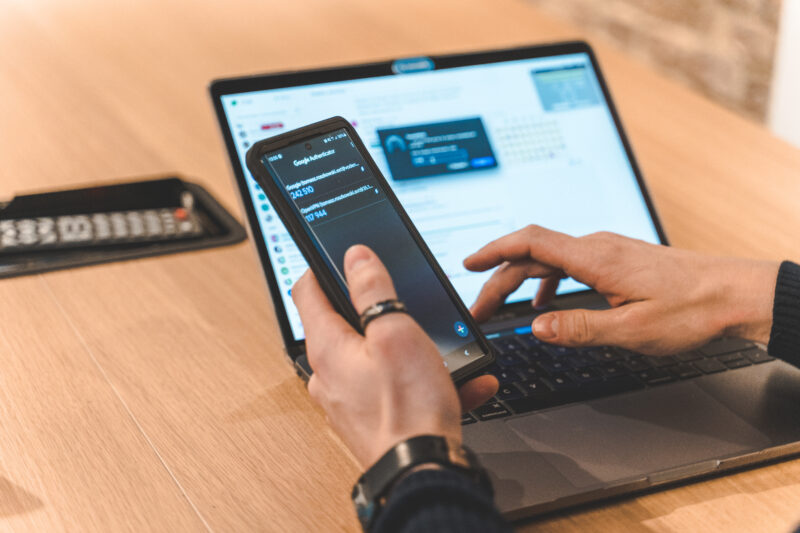
Effects
As a result of this project, our customer’s specialists responsible for posting offers benefitted from the ability and opportunity to check the expected performance of the offering. The specialists could now consider if the posting’s configuration was optimal.
Our Model used multiclass classification, which provided results in several buckets. The average accuracy of the results was above 70%.
The ability for specialists to use the new insights resulted in 7% lower CPC within 3 months of implementation.
-
7%
lower cost of CPC after 3 months
-
+70%
accuracy of classification model
Contact us
We will get back to you within 24 hours.