An international pharmaceutical company operating in several dozen countries, faced risk related to thousands of cross-border transactions, many of them with developing countries and in their local currencies. This, together with an suboptimal process of transactions’ preparation, exposed our customer to financial risk of fraud or mistakes.
Fraud and negligence detection
Challenge
Solution
An AI-based machine learning engine was recommended to detect transactions, which expose the company to risk higher than acceptable.
In the first stage of the project, data and process were analysed. It enabled the team to clean and prepare the data for further stages and understand the vulnerabilities in the process the customer was worried about. The main risk related to the process was caused by a possible erroneous or fraudulent change in the transaction list right before authorisation, such as currency change.
In the next stage, the team concentrated on further analysis of the data, as well as development and testing of models. Several models were shortlisted and presented to the customer. The selected model used unsupervised machine learning to identify clusters of transactions of fraudulent characteristics.
The model was used before the authorisation of the transaction list and records indicated as risky were flagged to be inspected manually by specialists. Feedback from specialists on the value of the insight was used by a regression model to score records more precisely. Ultimately, the system was supposed to flag just 50 riskiest records for further inspection per week.
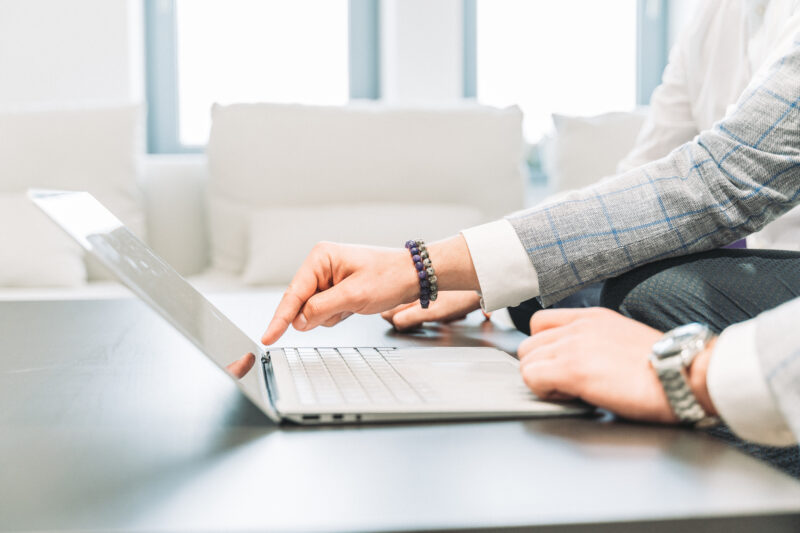
Effects
Implementation of the model significantly reduced risk as perceived by the customer in the area. According to feedback provided by specialists, 72% of flagged transactions were considered risky or for some other reason eligible for verification.
-
72%
accurately indicated risky transactions
-
significantly reduced risk in the area
Contact us
We will get back to you within 24 hours.