An importer and wholesaler of cars and spare car parts faced a challenge in managing its stock. Traditionally, individual points of sale (POS) were responsible for configuring products and placing orders. Too many of the decisions were made subjectively due to a lack of data and competencies required for a comprehensive analysis. As a result, the stock was rotating slower than its optimal pace.
Enhancing Stock Management
Challenge
Solution
We recommended an AI-based machine learning engine to indicate the expected amount of time needed for a product to find its customer depending on the location of the point of sale.
We began the project by analysing data that included the history of hundreds of thousands of products, which had rotated through our customer’s stock in the previous two years. The analysis revealed several data-related issues which were caused by imperfections in the data input process. Providing workshops with business specialists allowed all participants to gain insight into how to better prepare data throughout the process.
In the first stage of the project, data was analysed. It included the history of hundreds of thousands of products, which rotated through customers’ stock in the time frame of two previous years. Analysis revealed several issues related to data, which were caused by imperfections in the data input process. Workshops with business specialists allowed all participants to gain insight making it possible to prepare the data for further steps.
In the next stage, our team concentrated on further analysing the data, and developing, and testing models. Prepared models varied significantly. They included regression, classification, and clustering models, and they used data sets with varying levels of complexity.
The most valuable models ended up being classification models which indicated which products would be sold with the highest probability within a particular time frame. The selected model was implemented using BRAINHINT’s AI Farm software, which is a platform for managing machine learning engines and integrating them with business processes.
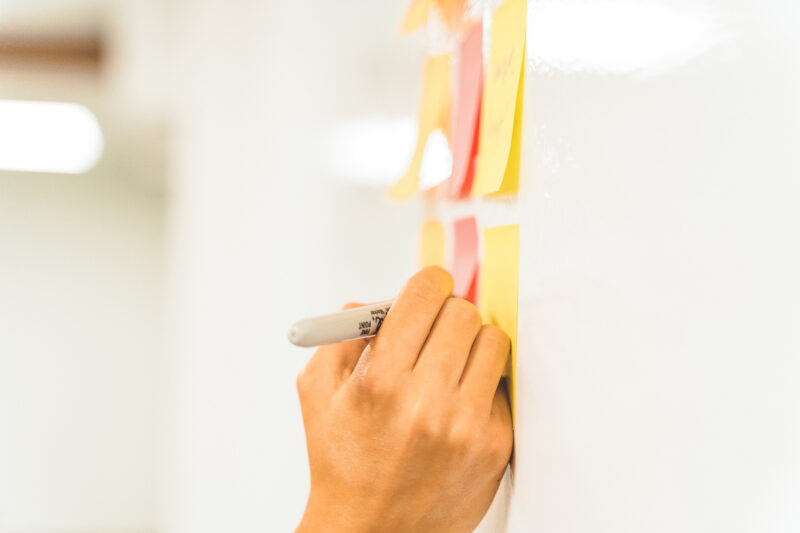
Effects
A sales representative can now ask for an estimate during the configuration of a product. Depending on the parameters indicated during the order placement, the model provides either suggestions to improve configuration by changing specific parameters or to resign completely from a given configuration.
Using the recommendations provided by the model resulted in improved stock turnover. Additionally, it decreased the number of products backing in stock by 34%.
-
34%
fewer products backing in stock for too long
-
improved general turnover of stock
Contact us
We will get back to you within 24 hours.